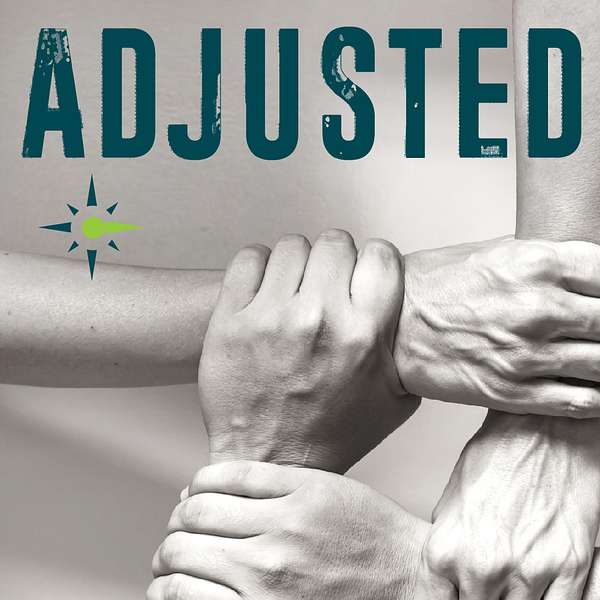
ADJUSTED
ADJUSTED
Data Driven Decision Making with Matt Murphy
On this Rebroadcast episode of ADJUSTED, we welcome a special guest, Matt Murphy, Vice President & Managing Actuary at Berkley Industrial Comp. Matt discusses all things data and decision-making: risks, rewards, and nuances.
Season 5 is brought to you by Berkley Industrial Comp. This episode is hosted by Greg Hamlin and guest co-host Mike Gilmartin, Area Vice President, Sales & Distribution, for Key Risk.
Comments and Feedback? Let us know at: https://www.surveymonkey.com/r/F5GCHWH
Visit the Berkley Industrial Comp blog for more!
Got questions? Send them to marketing@berkindcomp.com
For music inquiries, contact Cameron Runyan at camrunyan9@gmail.com
Hello, everybody, and welcome to adjusted. I'm your host Greg Hamlin coming at you from beautiful Birmingham, Alabama, and Berkeley industrial comp. And I'm excited to share with you today this rebroadcast. We did an episode over a year ago on data driven decision making with Matt Murphy. And Matt Murphy is our actuary. And I often joke that he's a bit of a unicorn and that a lot of the actuarial people I've met, speak at a level that I'm not able to understand with my common claims. Now, it's, and Matt does such a great job breaking down complicated ideas and being able to explain them to those who may not have a graduate degree in mathematics. And so I really loved this episode, because Matt talks about the importance of data driven decision making. I have the opportunity to work with Matt on a daily basis here at Berkeley industrial comp, and feel very privileged to have learned from him in my time here at Berkeley. And hope you do too, with this episode. So with that, we'll move to the episode. Hello, everybody, and welcome to adjusted. I'm your host Greg Hamlin coming at you from Berkeley industrial comp and Sweet Home Alabama, and with me as my co host for the day.
Mike Gilmartin:Yep. Michael Martin here, coming to you from Greensboro and key risks and excited to be heard, Greg.
Greg Hamlin:Awesome. Always glad to have Mike along with us for the ride. We have a special guest today, Matt Murphy, who is the Vice President and managing actuary at Berkley industrial comp. And we're excited to have him with us today. Matt, if you say hey to everybody. Yeah, hey, good. Good
Matt Murphy:to be here with Hambone the pot father himself and glad to be on here.
Greg Hamlin:Oh, we're great to have you. We're glad to have you back. So we're going to be talking about today data driven decision making. But before we get too far into that, Matt, I wanted to ask you, how did you get into the industry? Did you know when you were a small child and kindergarten that you are going to be a managing actuary of insurance company?
Matt Murphy:Yeah, good lord. No. So having been in the industry for a while, I find that the vast majority of us fell into insurance, right? None of us were really sitting there, like you said as kids imagining, studying for actuarial exams and the nice rewarding career insurance. So I actually started, I went to college and from New Orleans, so I went to college, in the Bronx, New York at Fordham University. And I was I was a student who was good at physics. In high school, I think a lot of people saw the subject difficult, whereas, you know, I felt like I could do the work. So I decided to make that my major in undergrad and graduated with a physics degree, headed back to New Orleans, where I kind of had this summer after I graduated to figure out what the heck I was going to do. Is it going to be grad school, which, which really was where I was sort of tilting towards. And then, you know, just serendipitously, my father went out to a dinner, and was sat next to the president of an insurance company. And, you know, basically said, Yeah, I got a deadbeat son at home and has no idea what to do with his life. The president of the insurance company said, Well, why don't you have him come in and do an interview? I think in hindsight now, this guy had just talked to him best. And they had impressed upon him. They needed an in house actuary. So I think he saw sort of my, my physics degree, my advanced math classes that I had already kind of taken and said, Hey, this, this might be our key to get that and you guys might not know, but the actuarial field is replete with these really onerous exams in order to get credentialed. So it was something I didn't even really know about the the industry or the actuarial professional field, until this and then I said, okay, yeah, I'll sit down, I'll take a couple of tests on these mathematical concepts. And, you know, I obviously did a little bit of reading up on it, and it saw that, you know, if you can pass these onerous exam requirements, it's a very lucrative and rewarding career. So, I jumped in from there and, you know, somehow made it through all the exams. And about, I'd say, 2017, mid 2017, I jumped from New Orleans and joined Berkley industrial comp, which was American mining at the time, but that's that's kind of my story, how I got here and obviously big aim steeped in data along the way.
Greg Hamlin:So how long? How long did it take you to pass all those exams? That seems like a nightmare?
Matt Murphy:Yeah, it is a nightmare. I was definitely, I failed a few of them. I know there's there's some folks who never fail a single one. So I guess it was good character building to learn to fail exams again. And I'd say I got my FCA S, which is the fellowship in 2016. So, all told, it was about it was about eight or nine years, from first to last, was pretty terrible.
Mike Gilmartin:My brain hurts just thinking about what goes on in your brain. I could just I can't comprehend what you think. I say that to Doug Ryan all the time, like you guys just think on a different wavelength. For listeners who maybe don't know, and I know we're gonna dive into the data aspect of it, but what do actuaries do? Like what what is what is your if you could some of your day to day job? What is it? Yeah, yeah,
Matt Murphy:I get asked this a ton, especially living in the southeast, where people have almost never even heard of the title, actuary. And, yeah, I like to just say, Hey, we're the glorified mathematicians of the insurance companies, right. So I would say there's really two main prongs for being a property casualty, actuary. And it's on the reserving side and on the rate making side. So, you know, we're in an interesting industry where, you know, a lot of times, you know, the cost of goods sold, right, so we, you know, you're you're serving up hamburgers, you know, the cost of the meat, you know, the cost of the buttons, the overhead etc, before you go in. Ours is an interesting industry where we don't know what the hamburger is going to cost when we sell it. So, so you really do need the actuary to come in and sort of estimate based off of historical data, and maybe industry data, if you're getting into a new line of business, what that cost is going to be so we're instrumental in setting those rates, or the premium that we're going to charge to get a risk to turn a risk on. The other side is the reserving side. I know, Greg Hamlin, and I talk quite a bit about this in our company, but it's basically once we've had the claim, okay, how much is it going to cost us now? How much do we need to hold up on our balance sheet as a liability to eventually pay this claim? At Ultimate is what we call it we say ultimate, and, you know, those that have been in claim to know that, you know, we may put up a reserve on a claim when we first see it, several things can happen to the claim, it can go south, we can get a favorable result. But but in the end, it's the job of the Actuary to take an inventory of all of our claims, or open claims and say, estimate, hey, this is what it's going to ultimately cost us at the end of the day, when it's all said and done. So those are the two main problems, I would say ratemaking. And reserving. And then, as Greg can also attest to, we also just fill in the random in between any sort of data requests from any any department, it usually falls on the actuary to figure out how to do that, or at least it does. And in my
Mike Gilmartin:experience, it does here too. And the fact that you like Greg, do math is a little scary.
Greg Hamlin:Yeah, you know, that's why I picked claims. Math is important. And we have some piece of that. But I'll say like, that wasn't my first strength, although you can get good at anything. If you put your mind to it, it would not. I definitely would not be able to be in your shoes, though, Matt, with the amount of high level math that you're doing on that end. So one of the challenges when you're trying to I assume, you know, as an actuary in a lot of ways you're trying to predict the future, or get a good idea of what that's gonna look like? What are the challenges that come along with that? For you to really be able to provide a good analysis of what to expect going forward?
Matt Murphy:Yeah, so I think it's a process. I think everybody likes to jump to the end really quick, right? And say, Oh, great. Why don't you just get out your crystal ball? Right? Why don't you augur the future for us here, read the bones, the tea leaves, right? It's not that simple. I think, in my experience, now, maybe when I jumped in, I thought that's what it was going to be, you know, it's all of a sudden, I'm just gonna get this data. I'm gonna tell you what's going to happen tomorrow. I think now I view it more as a process, right? So it needs several tiers of data and accompanies sort of relationship with data, needs several tiers to we can get to that sort of Holy Grail of predictive analytics. And I think the first thing to do is just to get a culture that enjoys data, right? That isn't scared of data that doesn't say hey, give it to the Okay, but actually, you know, I remember as a kid I knew I would have I love data because I would open up the sports page. And I would go to the stats pages, right? And see who's who's leading in homeruns. What are the records and all the sports? Who's got the most assists in the NHL? You know, it was it was things like that, that first drew me into statistics and stuff like that. And, and I think everybody has that sort of cursory curiosity. You know, even if you're not into math, I think you can find some stat nerds out there. And I think the first goal in this process is to get a few people in your company starting to want the data to see the data, right? Because, you know, first you got to measure it, but because because I do like this thing, you can't manage what you don't measure. Right. So measuring is, is is great, but I think, until you have that sort of second tier on top of it, which is actually analyzing it, and doing it at a frequency, you know, like you do, Greg, right. So we have, like dashboards that we spin up for Greg, he's looking at them, you know, at at bare minimum, weekly, but sometimes daily to see what's happening, right? If we get that sort of iteration, and I throw up data, you take a look at it, you what happens quickly is you find out when things are going awry, right? Or, Hey, this looks, this looks really wrong. And so I think that frequency and getting that culture to start churning over to opening that sports page every day, and telling me when blank values are coming over, for example, or Wow, this is 1,000%. That's way off. I've never seen higher than 20%. When you get that iterative feedback from people, and you get that culture that wants to look at these things, I think you're then at the next year, right now, now we're starting to feel comfortable with our data. We're starting to have some people ask for more, right? And get a little bit excited about it and say, Well, hey, Matt, this is cool. I really liked this part. It gave me this idea to go a little deeper, right. So I think what I see a lot of times is you have folks in the industry want to jump to the very end, like taking that flag out and running as fast as they can before the army is really in lockstep with them behind them. And I think it's much more powerful when you get this wave of, hey, we're coming out this as a group. And I think that makes these and that's not to say guys that I think we've made it to the very end at Berkeley industrial, I think we still have a lot further to go. And you know, as new technology comes out, it's a moving goalpost. But I think we have gone a long way in that middle tier, to getting a lot of senior leadership. Everybody in the company starting to say, oh, okay, I like the data, I want to see the data more. And I want it, I want that to push me to ask for more things. So I, at the end of the day, you know, I'm kind of rambling here, but I think Predictive analytics is and the crystal ball part is what everybody wants, and thinks, you know, hey, I'm gonna sign you know, I'll hire this data scientist, and I'm gonna have this tomorrow. I think it's much more of a process involved?
Mike Gilmartin:Well, I think you hit on something interesting. And it's something that I've talked to Doug ran about a number of times, but it's hard to start if your data is incredible to begin with. And there's so much in our industry, and I would imagine other industries, that is human input is what you get, people are putting into a claim, you get out what adjusters are, you know, clicking boxes and checking things. And those are some of the fields we utilize to drive some of our decisions and look at data and look at different reports. How do you as an actuary, how, maybe it goes back to culture, right, making sure everybody is in line with the fact that even the littlest thing you do affects the data that we have on the back end. But how do you make sure you get to the first step of your data? The incredible thing, that's a huge thing that you look at is great, this report is awesome. If it's right. So I think that that's where I find it fascinating what you guys can do, but how do you make sure data is credible? Yeah, okay, so
Matt Murphy:first thing, you know, to use the word credible, or credibility and actuarial circles. You know, we think of it slightly differently. Usually, when we're thinking about credibility, we're thinking of just volume, like, how much of it do you have? Are you trying to make inferences off of a small amount of data? That's not credible enough, even if the data are clean, right, completely clean? I think what you're more getting at Mike here is, you know, dirty data. And I'll give you a little anecdote on this. My, my first, my first actuarial conference, I mean, I don't know I might have had one exam under my well, I might not have even had any. But I remember going off to this with my CFO at the time. And I believe the title of the talk was like, bad debt, bad data anathema to actuarial. estimates or something like that. And what they did is this woman who was the speaker, asked everybody in the audience to just quick poll, like, what percent of your data would you say is clean? And here I am like, Oh, hey, I mean, my CFO, he's great. He knows, you know, he's preparing these reports, these reports are clean, they're great. And so I throw something out there, like 9095 or something. And then when all the results came back in, I think it might ended up as 10%. And it was gobsmacked by like, Oh, my goodness, while I'm keeping the data being clean, a lot more credit than they deserve. And now where I am, you know, 15 years into this industry? Oh, yeah, that 10% Maybe overestimated. So, great, great point to bring up there, Mike, that there's a lot of dirty data out there. And, and a lot of it is back to that feedback. mechanism I just talked about, like, we know, like it's been, it's been, it's a mantra, we got to go out there, we got to collect, we've got to hoover up all this data in insurance, right? Just Just get it log it. And I think a lot of times people don't see the point, right, or they see this is, man, I keep checking this box, it's onerous. Hey, guess what, I can actually skip this, I can leave this blank and go and look, if you're not managing that, if you're not analyzing that. And noticing folks do that, they will do it so long that you'll look back when you finally want to analyze that data in three years and say, Oh, my Lord, we never put anything in this field. And now we've got to, you know, go through, let's get somebody to try to backfill it all, which is never as good. So the important part, I think, too, like clean data, is constantly sort of analyzing it, and going over it and make sure that it's clean and giving those people who are inputting this data feedback. And that's not just you know, browbeating them to say, hey, you missed this, you missed this, but giving them a sense of the fuller picture about how every click, they're doing, you know, maybe giving them some of these summary results that are built off of those clicks. So they can see how important they are to the whole analytic enterprise. But yeah, data data's dirty.
Greg Hamlin:I man, I think you hit on something that was really important in that you talked a little bit about involving the people who are doing the work, because I think that's important for two reasons. And I think you caught you hit on those, but I want to make sure I emphasize them for people who are listening. One is, if the people who are going to use the data in the claims department or the underwriting department, have buy in, they're more likely to work to get the right data in there. And if you're asking them what they want, which is what you'd mentioned earlier, you're also more likely to have an end result that is functional and actually works for the people who are using it as opposed to building something in a vacuum, that you don't have the buy in it in. And so I think that's, that's really important. I've seen that in our department, and I appreciate the way you work with us on that piece to get it right. Because I think that makes a big difference. Yeah,
Matt Murphy:I think when you're analyzing too, you're gonna find that some of the data are extraneous, like, you know, yes, when we set this up, we said, I want you to do XY and Z. But if we're analyzing this later, and kind of saying, Wow, this is an onerous thing for these folks on the front lines to be putting in? And do you know what? Why are we even asking for this? We've got another way to get this data over here, right? So we're maybe being redundant or duplicating this entry. And so I think, looking back on it to say, you know, again, that feedback, that analysis to say, we don't need that. And the job isn't just we say this on day one, and you're gonna put all this information in, and don't worry about it. But that process can change as we continue to evolve and to go through and to comb through what's being swept up in the data. So
Greg Hamlin:I think that's great. So when you we may have some people who aren't familiar with predictive analytics, or what it even means, and how that applies to insurance. I know some folks have probably seen the movie Moneyball, which is maybe a good example of using statistics to drive outcomes. But when you when you think of predictive analytics, are you are explaining that to somebody who really has no experience with it. How would you do that?
Matt Murphy:Yeah, I mean, unfortunately, it's like one of those terms, like, you just take analytics, say, for example, or AI or predict it means something different to different people. I think to me, when I think of predictive analytics, I think of looking out of the windshield of the car, like we mentioned earlier and saying what's going to happen tomorrow? I think when people use analytics, it can be the the simplest thing, right? That doesn't involve any machine learning, but I think that it's gotten a lot of hype, not just in In the insurance industry in, you know, a myriad sort of use cases from, you know, image recognition, right. It's huge. Man the speech to text on your phone. I don't know if you guys have noticed, but in the last three or four years, it's phenomenal compared to where it used to be. Right. So I think all of these things are together, I think, how does it apply to insurance? It does rather well in what I would call, narrow use cases. So you know, that's, that's the one thing I think when people hear, say, AI or artificial intelligence, they're thinking that there's this. Watson, you remember IBM Watson, right? Brain that's going to win Jeopardy, but it was designed for one purpose, and it was just sort of win Jeopardy, right? You couldn't have it, give it a different task and say, Oh, Watson, you're so smart. Here's this other task, how are you going to do well on that? And they would say, Well, I'm not trained on any of it, I would do terrible. So I think people need to keep in mind that with AI and predictive analytics, where we stand today, there was a very famous example of the Google team, creating an algorithm called AlphaGo. I don't know if you guys have heard of this, but go. Apparently, in the world, you know, you've got chess, right? Which is, you know, more complicated than checkers. But then there's this, like, sort of supreme complicated game called go. It's very popular in Asia. And they had this Korean who was the world's best Go player, play against this algorithm? AlphaGo. Right. And I think a lot of people in the world thought that that was, AI was still very far away. And I think this was like 2016. So this is, this is about five years ago at this time. And I'm not sure if it was specifically, I think the the pro beat AlphaGo on the first run, and everybody was, you know, feeling good about themselves. But I think over the next few games, the algorithm just crushed the best human player. And a lot of people take these things and make headlines that oh, here we are, we've passed the singularity, right? It's, it's going to take over and, you know, I would caution that and say like, yeah, playing the game go. I mean, that's that's this thing isn't doesn't have a use case where we're Google can take this, and then immediately applied somewhere else, it's going to need tweaking, it's going to need a whole new set of data. So I think, when we talk predictive analytics, in insurance, in in some small, narrow use cases, I think it does really well like if you can say that it's kind of like the game go, right? If you're, if we're talking about like, what I've seen do pretty well is what we call like a claims triage model. Right? So in claims, we get a first notice of loss and right, and if we just have one rule, like all we're asking this algorithm to do is take these inputs and tell us is this claim going to be above X or below X and set that now where you set that level, it makes it a lot harder for this to get it right or wrong. But if you said that sounds like a low level, maybe if $2,500. So any claim that's you know, really going to be a loss, allocate allocated loss adjustment expenses, really, very little indemnity on it, it's it should be an open and shut case. It does rather well at finding these distinguishing these claims. I think that's a great use case. So, you know, on intake of these claims, we can shut these claims off to if this is going to be above X, hey, we can give this to a more experienced adjuster. We need humans in on this. If it's not, we might be able to program some automated rules to just deal with this claim, and get, you know, take this claim to the finish without any human intervention. I think that's possible. I think that's here today. I think when you start to get into, look, what we really want, what I think the Holy Grail is, is you give me an insurance application. And I know what the loss costs is like, I know what the cost of the burger is by what you gave me in your application. I think that is a little harder to do nowadays, even with, you know, all the datasets we have. Because, you know, this term gets thrown around in insurance a lot. We it's a fortuitous business. And I think a lot of people think the word fortuitous means fortunate it does not. It's more of happening by chance or random. And, you know, in our industry actuaries we love to talk frequency, which is how often does a claim happen, and severity when it does happen? How bad is it? I think when you get into areas that are very high severity, but don't happen a lot, low frequency, high severity. It's very hard for these predictive analytics to get this right, right. So we've got a roofer or you know, somebody who falls off a roof Well, we can write a lot of roofing accounts, how do we determine that one? policy that's going to pop and have a very complicated multimillion dollar fall claim. The truth is there's just too much randomness in it, that the inputs that we're giving the model, it can't it can't do it, right? I mean, it would have to have so many more inputs than we could ever fit on an on an application for insurance. So I think it along long winded here. I think Predictive analytics is here already. And it does great in some narrow use cases. Much better than I think we would have thought 510 years ago. But I think it still has limitations. I mean, you will, you will notice, sometimes, you know, your speech to text goes wrong. And it goes wrong big time, right? Or the Google algorithm that wants to predict, oh, hey, I can tell you what a cat is. I can see a cat in this photo a million times, right. But you put a cat in a swimming pool or something. And it's like, well, I have no idea what that is. Right. So there's certain little tweaks that we can do to fool them that makes a lot of people still say that it's it's still forming predictive analytics and machine learning for industrial applications. While very good, it's in narrow use cases, still has a long way to go. And actually, that's, that's exciting. You know, the to know that. There's still much more that we can do. But I think it will hit a limit, if that makes sense. Because of the fortuitous nature of some of these high severity, low frequencies. It's not going to be a black swan predictor. Right. You know? Well, I say that, but who knows? Who knows where we'll be in 10 years,
Mike Gilmartin:honestly. Yeah, I mean, I think you said a lot of things there. So I'm trying to digest all of it and figure out what my next question is. But so take, and it's interesting, you talked about predictive analytics, and any kind of being good for the one thing it's assigned to view. I've never really thought of it that way. And it's a really interesting way to think about it. So a lot of people I think, would ask, okay, take that small claim model, we have one key risk, I think you guys utilize one. How do you confirm? And this is just more for people to know, how do you confirm it's working? Or like how do you know you pick the right data elements to make it a successful model? Right, that seems and I was involved in how we pick our sticky risks, but it's still interesting, as you say, okay, these 12 data points will get us to a comfort level to say this claim is gonna be over x or under X. How does that process work? Yeah,
Matt Murphy:I mean, honestly, there's tests we can do with mathematics. And we can we can look for certain types of errors. Right? So, number one, if it is a small claim on our training set, we want to get it right. Right. So that's one way to look at it. Like if there's 100 of these small claims that we trained it on? How many did it find and say that that was small? Right? That's one thing. The other side is, how many does it? How many false positives does it have? How many did it say like, because I could, I could easily make a model that's, that can predict every one of the small claims, right? I can do it. Here's what I'll do. My model is a simple algorithm, every claim has a small claim. So I got a small claim. I know that sounds ridiculous. But you do have to take this to the extremes to sort of elucidate this. But the other thing is, how many false positives did you have? Right? And I think when you're talking about claims that can potentially get really big and bad. And Greg, you would know, like, wow, how did this claim ever get filtered off to this automated algorithm? With these inputs? You know, this is a multimillion dollar claim, we should have been on this, you know, from the first 30 minutes that it was in the door, I think that becomes the bigger test on something like the small claims, like, is it gonna miss? Which ones does it miss that goes above, right? So if there's two sort of ways to look at a lot of those classification problems, your sort of accuracy, your false positives, you know, that sort of thing. And mathematically, we can quantify those and talk them over with senior leaders, such as Greg and say, you know, what's your tolerance? You know, because if we want to use this, you're gonna have to have some tolerance for mistakes. I mean, let's be honest, we make mistakes as humans, sure. But it's, it's about drawing that line of where are we comfortable in knowing that there will be some mistakes here. But maybe it becomes it surpasses what a human could do. Still a little bit scary, right to take your hands off the wheel. But I think that's kind of the way you would you would measure this. And also it would depend, you know, we're talking about a classification problem here, right? Is it above or below those are those are nice and predictive analytics, but sometimes we're also asking for a What's the last cost, right? So what's the premium I need to charge on this policy? That's one we're well I never really going to get it right, exactly. But how far off was All right. So there's all sorts of different analysis that you can do to test these things, depending on what type of predictive analytic problem you're trying to do. So,
Greg Hamlin:we talked a lot about insurance, I know you're pretty plugged into how data is changing the way we live. And for those who maybe haven't had as much experience on the data side and insurance, what are some of the things you've just seen overall, that has changed the way that we live? Because of analytics?
Matt Murphy:Yeah, yeah. Good question. I think I've read some statistic recently, where, in the last two or three years, we've created more information than we had from the dawn of time, two, three years ago, right. So the first thing you need to know is we are just spinning off data everywhere. Each one of us is right, you look at all of your smart devices. We call it like the Internet of Things, all of these devices are putting off data, reading your phone, whether you know it or not, is pinging your location to all of your apps. Right. So we're, we are just shedding information at an incredible rate now, so that that I would say, right off the bat is the biggest thing that has changed is just there's so much more and with so much more data comes some trouble, right? Because now there's a lot of noise. Well, what's good, what's bad? I think it look if I was to talk to anybody who would be getting into this or thinking about data, what I would say is like, there are some, there's some sexy parts to it, right? Like when you when you put up this visualization where you put up your model results at the end, and you show people the end product, what you don't see there is like that is the last 5%. You know, it's like an iceberg thing. Most of your time is done, like is spent with the menial, I gotta clean this data. Oh, my gosh, can you believe this? Why are these results coming in here? Look at all these outliers. And so before you can even get to the last part, right? Which becomes so much harder when you have so much more data if there's just a sea of it. Now, how do I clean it, transform it, mutate it, loaded in and make sure it's all in the right hoppers before I do my analysis. I think that takes up most of the time. And and I think now with, you know, the surfeit of data we have out there that is that much harder to do. Now, we do have technology that's coming along and making things a lot easier to I mean, just, you know, I don't know if you guys have heard of Moore's law, it's a famous thing where basically, I think it's the number of transistors that will fit on a computer chip, you know, doubles every year, right. So we can progressively get more and more smaller and smaller to where in the 60s MIT, I think had the first computer it was took up a whole warehouse. Now you've got so much more power in it in your pocket, right? I think the same thing is happening for data and the ability to deal with large amounts of data and to like sort of automate a lot of this cleanup process. So that is happening in lockstep. But you got to beware, right? Because there's so much more out there. If you're not using these things properly, you could get some very, what we would say spurious results, that that aren't what you what you imagine and are purporting to be something but well, you made one mistake here, you missed a comma. And that made the result look very different. So I think the stakes have gotten higher, with with doing sloppy, sloppy data work, just because of how much more information there is out there. And there's more. I mean, there's gonna be more this year. There's gonna be more next year. I don't see that pace slowing down, similar to sort of the Moore's Law of, Hey, man, we keep making double. You and I know it, I mean, you probably have some, you know, Amazon, what is it Alexa at home or echo? Whatever it is for the Google. I mean, it's recording, all of it, right? being stored. I mean, that's a scary thing. And I think I should touch on this too. The other very scary thing about having all this data are privacy issues, right. And especially with respect to how I deal with it, right, I'm dealing with a lot of data. And some of this is personally identifiable information, right. And so we need to be very careful, we need to be very good stewards of the data we're given and adhere to all of these, you know, we've got regulations coming out, really, I think Europe has led the way in data privacy and governance, but California is right there in New York is right there. And so that's very important with all of this data becomes a lot of responsibility to use it in an ethical way. And with respect for for individuals privacy, so I think that's the other thing that has changed a lot, Greg is, you know, a lot more consideration With respect to privacy. Awesome. So
Greg Hamlin:when you are working with people who haven't used a lot of data, what are some of the challenges in getting people to adopt the use of data in their decision making? You know, I know, maybe for somebody who's recently out of college that's maybe not as big of a hurdle. But somebody who's been doing insurance or been involved in the industry for 2030, maybe longer? What are some of the challenges in getting others to adopt the use of data and their decision making?
Matt Murphy:Yeah, I think that's a great, great question. I have seen a lot of a lot of different folks in my time in the insurance industry. I think the thing I think of immediately when you say that is bias and narrative bias, I think the thing we all need to know is that we're human beings, and we are full of biases, right? We may think we're special but no, our human brains are broken in slight ways. That that make us very biased. So I've seen a lot of this, Greg, I've seen, say, folks who say, you know, the data can't tell me anything I don't already know. And I think what you see in a lot of those folks, is they do what you call cherry pick data, right? If this confirms my narrative bias, right? If this adheres to this narrative, I'm going to take that data. If it doesn't, I pretend it doesn't exist, and put it away. I think we talk a lot about at Berkeley industry about evidence based decision making. And I think that's where you have to start. And honestly, there's a humility to knowing that you make mistakes in your brain, right? We have intuition and intuition is amazing. The human brains intuition is simply outstanding, it's works well, 95% of times 5% of the time, it gets you into a lot of trouble. Right. And I think being aware of that is great. And as you said, I think I think the younger folks in this industry are much more receptive to evidence based decision making, as you know, counterintuitive as that may sound, some of the older folks in the industry have been doing this for 30 years. And so they've gathered what we call a lot of priors. Okay, this happened this way. Back when I first started, I'm going to see this again. And that's not always the case. And I think that that can be very dangerous, when people try to use data only to confirm their biases. And not just say, start off with a clean slate of like, okay, well, first, what did the data tell me? Right before I start crafting a narrative? Let's look at the evidence first. I think that's big. So so how do you get there? It's tough sometimes. I think. I think what you do is you try to get people like I said, in these tears, right? I'm not going to take you all the way to a this model is going to give you the underwriter has been doing this 30 years the answer? I think we need to walk that underwriter through every tear in this process, before we get to the right, get them comfortable with you know, just analyzing the data that we do have or the history right, looking at the rearview mirror as opposed to the front, right. I think if we get them along, we can we can start to move them towards the evidence based decision making. That sort of suppresses those those biases. But hey, I'm human, I do it too. So honestly, I fall for a lot of these still. And I know that I need to watch out. So so it's tough. I do think that the younger younger minds are more receptive to it, though. To your point, right.
Mike Gilmartin:First of all, sexy data is a new term I'm going to use everywhere. So that's done like I'm very excited. You're welcome. Well, you have a couple things that I find I find really interesting. And I, I've been kind of one of the guinea pigs and curious and getting involved in the data and kind of some of our data visualization tools and how far those have come. But I think you said some people can spin data in a way that either confirms or negates an argument. And that can get kind of scary, right? Instead of looking at the data for what it is. They kind of look at it as Yeah, confirms my story, or no, it doesn't. Or maybe they're not even looking at the right thing. And one of the things that Doug and I talked about all the time is, is what's your is the data that you're visualizing, actually telling the story you want it to tell meaning? Is it accurate? Like are you actually if you throw up a graphic, is it actually driving to the graphic you're trying to show? And do you have the right elements in there to get there? And I think so many times nowadays, there's so much visualization out there and there's so much data, you have somebody put up a graph or a visual, it has like 700 different things on it. And it's like I don't know what you're trying to tell me. I'm not really sure what the story is being told. I just don't know, how do we how do we continue to manage that as we get more and more data involved in the decisions we're making on a daily basis? Because sometimes, I mean, there's just too much. So I didn't know from an extra standpoint, how do you kind of dumb it down so that what you're trying to show tells the story you want it to? If that question makes any sense at all? Oh, no, it's
Matt Murphy:a great question, Mike. And I see it all the time. So all you need to do I think USA Today does a great job of giving you terrible visualizations that may look pretty, but do not confer to the audience any sense of what they were trying to say. It's everywhere. It really is ubiquitous. I see it all the time, where somebody's using the wrong visualization, right? Or they're trying to display something, but they use the wrong type of chart, right? Hey, this is a time series you should really be using alive, even though and yes, it's a big up the edge of again, sexy. Some of these visuals are like a stat. I've seen a lot of them. And I'm like, wow, that looks really cool. And then just like you I spent 30 seconds, I'm like, but I have no idea what they're trying to tell me in this visual. Other than that, look how cool this looks. So I think that that, that is definitely something that we have to, we have to measure against, we have to weigh the pros and cons because on the one hand, the marketing is great. And honestly a great visual can bring in an audience well, and get them to the next level of understanding the data. But at the same time, you can confuse a lot of people. And it's very difficult. If you're an actuary, say like me or Doug, who's like, oh, yeah, you guys, you guys just don't get this way. Come on. It's three axes here. You don't see the Z axis. Like no, some people don't see it in the same way. So I think it is kind of level setting, putting yourself is in your audience shoes, right? If it's the USA Today, readership, you better you better get this lowest common denominator, right, we might just want to use a simple bar chart. And that's it. And I've seen some of the best examples of, you know, here's this great sophisticated one. And here's what you really should have done. And it looks so simple. And it's lost all of that appeal of visually stunning. But man, if it doesn't get the point across is so well, and so cogently. So I think it's a it's a trade off of you know, because we do still want to bring in audience members, and we want to wow them. But I think you're right, the Prime Directive here is to give them the right data. And if we go off of that, and anything we can do underneath it, to make it look better, cool, approachable. I think that that's kind of the next layer on top. But yeah, I see it all the time. Mike is a great, great question. And, you know, some folks, you know, just start using a lot of, you know, words, a word soup to describe something. And, you know, you really could have said it in three words that that the layman could understand. So, great point. You know, it's a tough trade off, I think between between the appealing look and sounding smart, and then actually getting something across to your audience that they understand.
Mike Gilmartin:That's what I'm doing all the time, the level of chart that he understands versus the level of chart that I understand things, but I think it's just a great point, when we're talking about data driven decision making it to me, the people that we put this in the hands of are not actuaries, they're not folks that generally spend eight hours of their day digging into data and really understanding what goes into it, they need a simple, kind of, here's what the information is. And now I need to I need to act on that. And I think it's just sometimes we missed that mark of the folks who are actually gonna utilize this data or this chart, or, you know, this visual needed as simple as possible, because that does drive the next decision they're gonna make, I just think it's, it's an important point to make. And because you see it all the time, everywhere of like, I don't even know what this is telling me. And I'm not sure I understand that. And I'm a fairly smart guy. So
Matt Murphy:yeah, I think that's definitely one of the skills, you know, that I wouldn't have thought of coming into this industry. As an actuary, you gotta have, right I mean, a lot of people think of the actuaries disease, you know, eggheads, who are, you know, nerd out about everything and could get really narrow and focused on something and go to these deep levels. But I think what makes you a really good one is if you could come up to the level, know your audience, and convey complicated complex information to a regular Layman and do it to where they come out of it being like, yes, maybe I didn't go completely deep and and understand everything you were talking about, but I got the gist of it. I think. I think that's a really important skill to have, especially when you're dealing with data even if you're outside of actuarial.
Greg Hamlin:I agree completely. And and I think, you know, from my experience, what That's really added a lot of value to my job is the ability to use that information to make everybody's jobs easier, make better decisions, you know, I think about some of the things we've worked together on, you know, from managing plane counts to making sure they're balanced to making sure the right claims are with the right people to, you know, be able to dive deeper into some of our more difficult claims and analyze them and in new new ways, has been game changing. And so for those who are thinking about a career in and data, I think there's a bright future. And I think there's so much that can still be done. So I want to thank you, Matt, for joining us today and going over this topic with us. And really appreciate all that you've added in that. And I hope that for those who are listening that you'll continue to join us for future podcasts releasing every two weeks. If you can't get enough adjusted in your life, check out our adjusted blog for our resident blogger Natalie dangles. bootstrappers it drops on the opposite Monday of the podcast, and it can be found at WWW dot Burk in comp.com. One thing we're doing different if you have questions regarding this episode, or previous episodes, we'd love to hear from you. So please send your questions via email to marketing at Burke in comp.com. We read everything you send us and we'll try to address questions and future episodes of the podcast. So especially if you got some questions for Matt, or on this podcast or previous ones, send them our way. And if you liked your Listen, please give us a review on Apple's podcast platform. We want to express Special thanks to Cameron Runyan for our excellent music. If you need more music in your life, please contact him directly by locating his email on our show notes. And thanks again for all your support. And remember, do write think differently. And don't forget to care. That's it for today, folks.